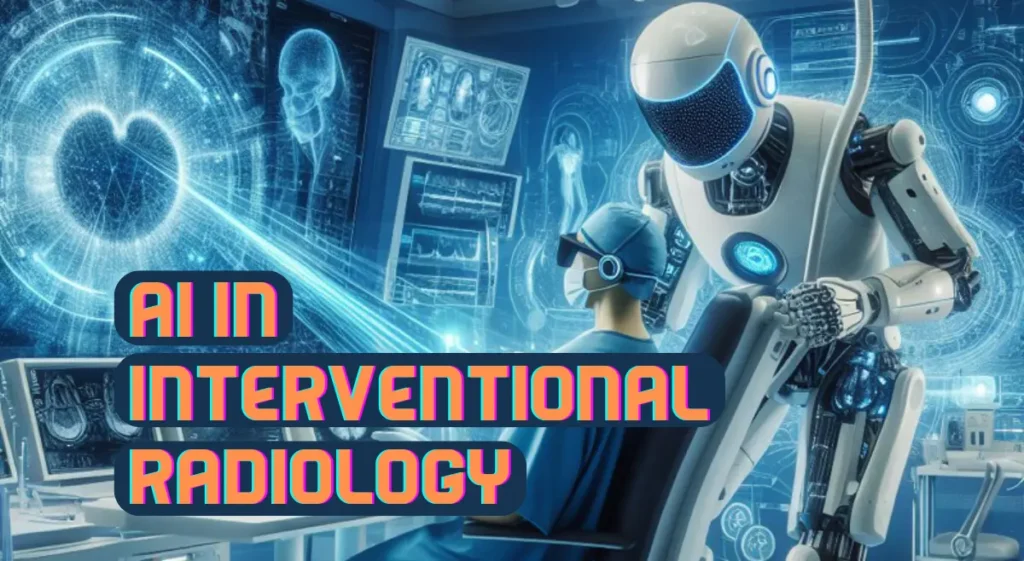
The Intersection of AI and IR
Interventional radiology, known for its minimally invasive techniques guided by medical imaging, has embraced AI to further its capabilities. AI in interventional radiology represents a synergy that enhances precision, improves patient outcomes, and streamlines clinical workflows.
Transforming Imaging with AI
- At the heart of IR lies medical imaging, and AI has revolutionized this domain. Through advanced algorithms, AI enhances image quality, facilitates faster interpretation, and aids in the accurate detection of pathologies.
Navigating Procedures with Precision
- AI technologies offer real-time assistance during IR procedures. By processing imaging data instantaneously, AI guides radiologists in navigating instruments to the target area with minimal invasiveness and higher accuracy.
Deep Learning: The Core of AI in IR
Deep learning, a subset of machine learning, utilizes neural networks to interpret complex medical data. Its application in IR is groundbreaking, offering:
- Enhanced Lesion Detection: Deep learning models excel at identifying and classifying lesions, often surpassing human accuracy.
- Automated Segmentation: These models streamline the segmentation of organs and pathological structures, crucial for planning IR procedures.
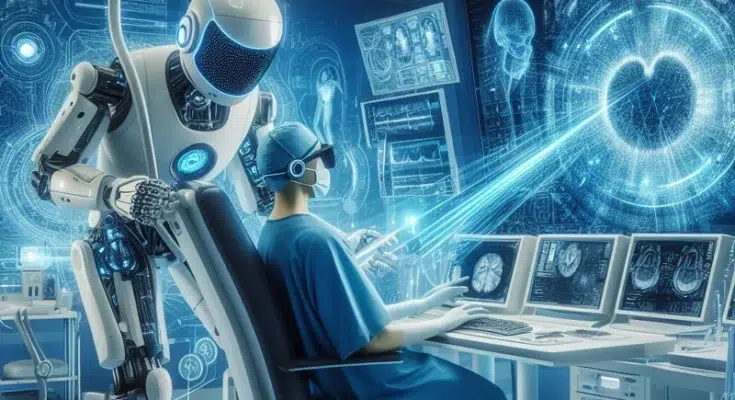
Top 10 Benefits of AI in Interventional Radiology
- Enhanced Cardiac Imaging: AI improves visualization in cardiac imaging, aiding in the diagnosis and treatment of heart conditions.
- Brain Tumor Classification: AI classifies brain tumors with high accuracy, facilitating early intervention.
- Detection of Vertebral Fractures: AI assists in spotting vertebral fractures, often difficult to detect in early stages.
- Alzheimer’s Disease Detection: AI aids in the early detection of Alzheimer’s disease, allowing for timely care.
- ALS Diagnosis: AI contributes to the diagnosis of ALS by analyzing patterns in imaging data.
- Radiology Reporting: AI streamlines radiology reporting and data-related tasks, enhancing workflow efficiency.
- Breast Cancer Detection: AI improves the detection of breast cancer, leading to better survival rates.
- Dose Optimization: AI optimizes dosing in imaging procedures, reducing patient exposure to radiation.
- Pneumonia Detection: AI accurately detects pneumonia, a critical capability in emergency medicine.
- Large Vessel Occlusion (LVO) Detection: AI detects LVOs, crucial for stroke diagnosis and treatment.
The Impact of AI on IR Outcomes
The integration of AI into IR has led to improved patient outcomes. AI-driven interventions are characterized by their accuracy, leading to reduced complication rates and quicker recovery times.
Predictive Analytics in Patient Care
- AI’s predictive analytics play a crucial role in personalizing treatment plans. By analyzing patient data, AI can forecast the efficacy of interventions, allowing for tailored treatment strategies.
Post-Intervention Monitoring
- AI’s role extends beyond the procedure room. Post-intervention, AI systems monitor patients for signs of complications, ensuring prompt responses to any adverse events.
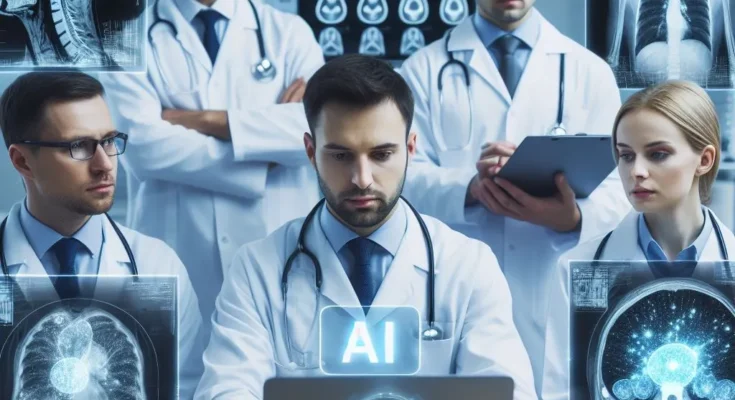
The Ethical Dimension of AI in Interventional Radiology
As AI becomes more entrenched in IR, ethical considerations come to the fore. Issues such as data privacy, algorithmic bias, and informed consent must be addressed to maintain trust in AI-assisted healthcare.
Looking Forward: The Future of AI in Interventional Radiology
The potential of AI in interventional radiology is vast, with ongoing research and development promising even more sophisticated applications. Future advancements may include fully autonomous IR procedures and complex predictive models for personalized medicine.
Commonly Asked Questions about "AI in Interventional Radiology"
- AI is utilized in interventional radiology to assist with image interpretation, treatment planning, and procedural guidance. Machine learning algorithms analyze medical images to aid in diagnosis, identify abnormalities, and optimize treatment strategies.
- Various machine learning algorithms, such as convolutional neural networks (CNNs), recurrent neural networks (RNNs), and deep learning models, are employed in interventional radiology. These algorithms are trained on large datasets of medical images to recognize patterns and make predictions.
- Interventional radiology procedures utilize a range of technologies, including fluoroscopy, ultrasound, CT (computed tomography), MRI (magnetic resonance imaging), and angiography equipment. These imaging modalities provide real-time visualization and guidance during minimally invasive procedures.
- The future of AI in medical imaging is promising, with advancements expected in image analysis, diagnostic accuracy, and personalized treatment planning. AI algorithms will continue to evolve to assist radiologists in detecting subtle abnormalities, predicting patient outcomes, and optimizing clinical workflows.
- Artificial intelligence is revolutionizing radiology pathology by automating tasks such as image interpretation, lesion detection, and quantitative analysis. AI-powered software enhances radiologists’ efficiency, improves diagnostic accuracy, and enables personalized treatment planning for patients.
AI streamlines radiology workflow by automating routine tasks, prioritizing urgent cases, and providing decision support to radiologists. AI algorithms analyze medical images, extract relevant information, and generate structured reports, leading to more efficient and standardized patient care.
AI facilitates precision medicine in radiology by integrating patient data, medical imaging, and genomic information to tailor treatment strategies to individual patients. AI algorithms analyze complex datasets to identify biomarkers, predict treatment response, and optimize therapeutic interventions.
Despite its potential benefits, integrating AI into interventional radiology practice poses challenges such as data privacy concerns, regulatory compliance, algorithm validation, and interoperability with existing healthcare systems. Collaboration between radiologists, data scientists, and regulatory authorities is essential to address these challenges and ensure the safe and effective implementation of AI technologies.